Our team designed and executed a migration plan that moved ten years of historical data along with the latest daily feeds from different upstream systems to an Azure data lake. We configured and scheduled extract, transform, and load (ETL) jobs to summarize data and send reports to business users through Power BI and Azure SQL Reporting.
Our quality engineering team assisted the company in the validation performed during data migration and conversion. We also performed business validation on revenue accounting system data. The team validated business rules applied to the data and conducted data sampling testing for migrated and converted records in Azure Databricks via Structured Query Language (SQL). Using SQL in our solution was ideal because, at this stage, there was no UI (User Interface) or API involvement.
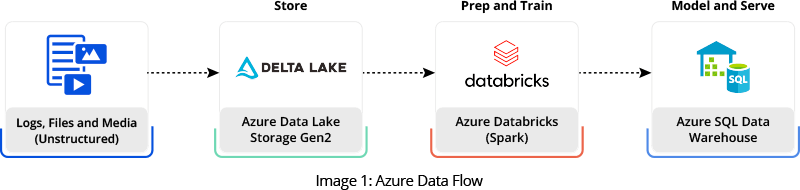
Image Credit: Tutorial: Extract, transform, and load data by using Azure Databricks
During our preliminary analysis, we explored and proposed two proof-of-concepts. One used ‘Great Expectations,’ and the other leveraged ‘Tricentis Tosca Data Integrity’ (Tosca DI).
Great Expectations is an open-source Python library that helps produce assertions for PySpark data frames. This would have been a good choice as we built our software on Azure Data Lake. However, subsequent investigation uncovered some shortcomings.
Tosca DI successfully fulfilled all our automation needs, enabling us to compare a high volume of data on an enormous scale in a reasonable timeframe. The company was already utilizing Tosca for other applications. Consequently, the company decided upon Tosca and we recommended the Tosca DI module, a tool purposefully designed to meet data integrity testing needs.
Our team developed automation scripts using SQL in Tosca DI to address the challenges we encountered. These scripts effectively identified numerous functional defects that functional testing had previously missed due to the overwhelming data scale. These automation scripts became indispensable in regression testing, which was manually impractical due to the data volume.
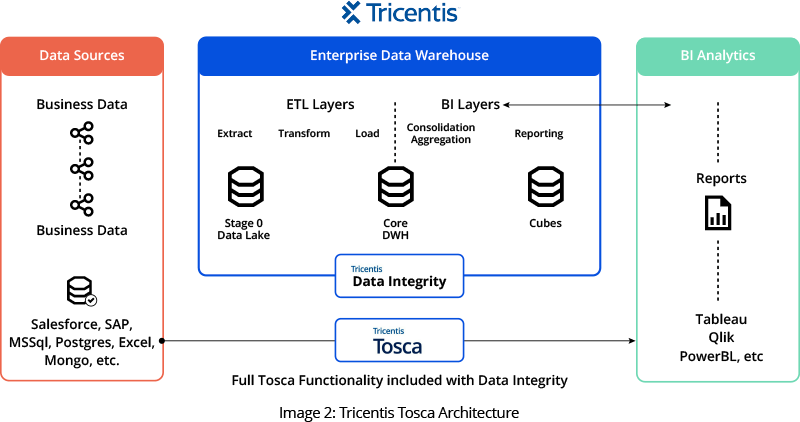
Image Credit: Tricentis Tosca
Below are some key results we achieved through automation:
- Metadata testing: This allows the company to obtain information about the data within the schema, for example, the column names, the data type of a column, and constraints imposed on the data. The new system incorporates a rich set of additional validation that includes checks on the data type, length, constraint, and naming conventions.
- Data completeness testing: All expected data is extracted from the source and loaded into the target without loss or corruption. The company can confirm the row count, aggregate column data values, validate primary keys, and perform a full-value comparison validation. In addition, they can now monitor missing setups.
- Data quality testing: The new system allows us to check that the business rules and logic have been applied to the ETL pipeline. Quality tests detect duplicate data, confirm that business rules have been applied, and perform data integrity validation.
- Draft run: Our solution lets the company view journal data before the final posting to the company’s Oracle accounting systems.
- Fully automated auto-accept process:The new system validates all the daily store data per business requirements.
- Scalability and futureproofing:Testing and validation automation using Tosca DI helped validate millions of upstream records in minutes.